
Distinguished Lecturer
Dr. Qi-jun Zhang
Distinguished Lecturer
Prof. Qi-jun Zhang to present “Emerging AI/Machine Learning Technologies for Analysis and Optimization in High-Speed/High-Frequency Packages and Systems” on Wednesday, June 25th, 4:00pm – 5:30pm (EDT).
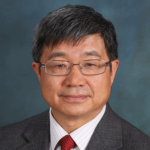
About Dr. Qi-jun Zhang
Term 2025-2026
Chancellor’s Professor, Department of Electronics, Carleton University
qijunzhang@cunet.carleton.ca
Dr. Zhang received the B.Eng. degree in EE from Nanjing University of Science and Technology (Nanjing) in 1982, and the Ph,D. degree in EE from McMaster University (Hamilton, Ontario) in 1987. He was a researcher engineer in Optimization Systems Associates Inc. (Dundas, Ontario) during 1988-1990 developing advanced microwave optimization software. He joined the Department of Electronics, Carleton University in 1990, where he is currently a Chancellor’s Professor.
Dr. Zhang’s research area is AI/machine learning, and optimization for designing high-speed/high-frequency components/packages and systems, which are building blocks of computers, wireless and wired systems in telecommunications, internet, and intelligent and autonomous systems.
Dr. Zhang advanced the theory and practice of microwave active and passive computer-aided design with innovations in linear and nonlinear device modeling and circuit optimization for which he received the IEEE Fellowship in 2006.
Dr. Zhang is one of the pioneers of neural networks and machine learning for microwaves which started in the early 1990s. Dr. Zhang’s continuous innovations in this area for over 30 years have established many research milestones, and contributed to the substantial development of the area. He authored (with Prof. K.C. Gupta) the first book of the area (Neural Networks for RF and Microwave Design, Boston, Artech House, 2000), and developed the first software (NeuroModeler, 1998) of the area. His neural network based modeling technology was adopted in industrial projects such as the Advanced Embedded Passives Technology Consortium (2000-2003) funded by the US Department of Commerce and the National Center for Manufacturing Sciences (Michigan, USA). In 2012, neural network based transistor modeling technology pioneered by him and his student became a primary feature of Agilent/Keysight IC-CAP software, the microwave industry’s dominant modeling tool. Over 360 technical papers archive his pioneering contributions. Example of publication: Q.J. Zhang, K.C. Gupta and V.K. Devabhaktuni, “Artificial neural networks for RF and microwave design: from theory to practice,” IEEE Trans. Microwave Theory Techniques, vol. 51, 2003, pp. 1339-1350.
Dr. Zhang’s paper with his former PhD students F. Feng, W.C. Na, J. Jin, J.N. Zhang, W. Zhang, titled “Artificial neural networks for microwave computer-aided design: the state of the art,” IEEE Transactions on Microwave Theory and Techniques, vol. 70, no. 11, pp. 4597-4619, Nov. 2022 became hugely influential. The paper is ranked the #1 most frequently accessed paper in a given month among all papers of IEEE Transactions on Microwave Theory and Techniques, for each and every month for 11 months in a row since its publication in November 2022. At the time of writing this paragraph, the latest monthly statistics (October 2024) shows that this paper is currently still the #1 most frequently accessed paper of the IEEE Transactions on Microwave Theory and Techniques.
Talk 1: Emerging AI/Machine Learning Technologies for Analysis and Optimization in High-Speed/High-Frequency Packages and Systems
Abstract: AI and machine learning are unconventional technologies with unique capability to address challenges in electromagnetic-based analysis and optimization in high-speed/high-frequency electronic components/packages and systems. With phenomenal progress in electromagnetic based computation algorithms, along with dramatic changes in the computing environment, high-fidelity electromagnetic models are now an important part of high-speed/high-frequency electromagnetic design automation. However new design challenges continue to rise. Electromagnetic structures and circuits are becoming more complex, and frequency is getting higher. More sophistication in multiphysics modeling and design are becoming increasingly necessary. Meaningful design problems easily become computationally prohibitive.
In this talk, we present AI/machine learning technologies for electromagnetic /multiphysics based modeling and optimization, and their applications to signal/power integrity analysis of highspeed/high-frequency electronic packages and subsystems. We will highlight emerging directions of knowledge-based, cognition-driven design. Incorporating domain-specific design knowledge/engineering equations into artificial neural networks, knowledge-based and deep-learning based computational technologies are producing fine-grained modeling and design solutions for problems which are otherwise computationally very expensive. New formulations of inverse neural network training algorithms allow instant solutions to electromagnetic inverse modeling problems addressing the technical challenges of non-uniqueness in inverse modeling. Emerging machine learning structures and optimization algorithms for electromagnetic based design and signal/power integrity analysis will be discussed.
Talk 2: Quantum Computing for Machine Learning, Electromagnetic Simulation and Applications to EMC Analysis
Abstract: Quantum computing attracts increasing attention from the computation community in recent years. In certain cases, quantum algorithms has the potential of exponential speedups over their classical counterparts running on classical computers. Quantum computing opens many new opportunities for solving large-scale problems, and simulating complex physical systems. In this talk, we explore quantum computing for AI/machine learning oriented computations, and application of quantum computing in solving electromagnetic problems. Preliminary exploration of quantum computing for solving electromagnetic field simulation problems have been conducted by researchers, using such as Transmission Line Matrix (TLM), Method of Moments (MoM) and Finite-Element Method (FEM) formulations. We present two types of quantum computing approaches for electromagnetic simulation, one is a complete quantum computing approach, utilizing Harrow–Hassidim–Lloyd (HHL) algorithm; another one is a hybrid classical/quantum computing approach utilizing Variational Quantum Algorithm (VQA). New formulations of electromagnetic equations such as FEM equations, into quantum-compatible format will be described. Methods to prepare electromagnetic field excitation vectors into quantum states will be described. Methods to determine various hyperparameters in quantum computing based electromagnetic simulation will be described. Potential applications for EMC analysis will be explored.